AI DRIVEN BI - ESSENTIAL AI AND BI TERMS YOU SHOULD KNOW
- Jennifer
- Sep 9, 2024
- 3 min read
When I first ventured into the world of business intelligence, I often found myself completely lost during conversations filled with buzzwords like ML, AI, Data Warehousing, and many more. I remember thinking, "What on earth are they talking about?" I turned to the internet for help, hoping to make sense of these terms, but instead, I felt even more overwhelmed by all the technical jargon.
That’s why the TA Insights team came up with the idea to create this guide—for people like me, who felt confused, or for those who are simply curious. Below is a list of 30 essential terms you're bound to encounter when discussing BI and AI. Think of it as your very own simplified AI/BI mini dictionary.
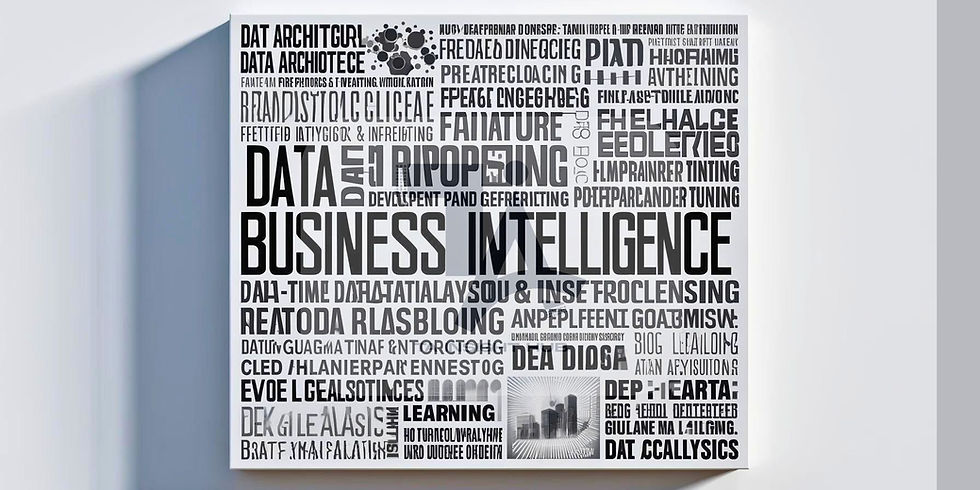
Let’s dive in!
AI Governance: The rules and guidelines for using AI responsibly.
AI Model Selection and Training: Choosing the best AI model (A system trained to recognize patterns and make decisions based on data) and teaching it to perform a specific task using data.
Artificial Intelligence: A computer system that can perform tasks that normally require human intelligence, like decision-making or recognizing patterns.
Big Data: Very large and complex data sets that are too difficult to process using traditional methods.
Business Intelligence: The process of using data to make informed business decisions.
ChatGPT: A large AI language model created by OpenAI that can understand and generate human-like text based on the input it receives.
Data Analytics: The process of examining data to draw conclusions and make decisions.
Data Architecture: The structure and design of how data is stored, managed, and used in an organization.
Data Cleaning: Fixing or removing incorrect or incomplete data to make it ready for analysis.
Data Pipelines: The process of moving data from one place (like a database) to another for analysis.
Data Preprocessing and Integration: Preparing data (like cleaning and organizing) and combining data from different sources to use in analysis.
Data Science: The field of study that uses methods, processes, and algorithms to extract insights and knowledge from data.
Data Storytelling: Presenting data in a way that tells a story or explains a concept clearly.
Data Visualization: Showing data in a visual form, like charts or graphs, to make it easier to understand.
Data Warehousing: The process of collecting and storing large amounts of data from different sources in a centralized location (called a data warehouse). This data is organized and structured for easy access, analysis, and reporting to help businesses make better decisions.
Deep Learning: A type of machine learning that uses complex algorithms to mimic the human brain's way of processing information.
Dynamic Dashboards: Interactive dashboards that update automatically with real-time data.
Ensemble Learning: Combining multiple AI models to get better results.
Event Stream Processing: Handling and analyzing continuous streams of data in real-time.
Explainable AI (XAI): A type of artificial intelligence designed to make its decision-making process understandable and transparent to humans.
Feature Engineering: Creating new, useful data from existing data to improve the performance of an AI model.
Hyperparameter Tuning: Adjusting settings in an AI model to make it work better.
Internet of Things (IoT): A network of physical devices (like smart appliances or sensors) connected to the internet, which can collect and share data without human involvement.
Machine Learning: A branch of AI where computers learn from data without being explicitly programmed.
Model Development and Training: Creating an AI model and teaching it to recognize patterns using data.
Natural Language Interfaces and Voice Analytics: Using spoken or written language to interact with computers and analyze what people say.
Natural Language Processing (NLP): AI that helps computers understand, interpret, and respond to human language.
Predictive Analytics and Forecasting: Using data to predict future events or trends.
Real-time Data Analysis & Insight Generation: Analyzing data instantly to provide immediate insights
Time Series Analysis: Analyzing data that is collected over time, like stock prices or weather data.
Comments