DATA MINING IN ENTERPRISE RISK MANAGEMENT
- Jennifer
- Oct 28, 2024
- 5 min read
Updated: Nov 6, 2024
In today’s fast-paced business world, managing risks effectively has become essential for maintaining organizational resilience and fostering growth. Enterprise Risk Management (ERM) provides companies with a structured approach to identify, assess, and mitigate risks across all levels. Yet, traditional methods of risk management, often reliant on historical data and qualitative assessments, struggle to keep up with the increasing scale and complexity of modern data. Here, data mining steps in as a powerful ally, offering advanced, data-driven techniques to enhance ERM by uncovering hidden patterns, trends, and correlations that might signal future risks or opportunities.
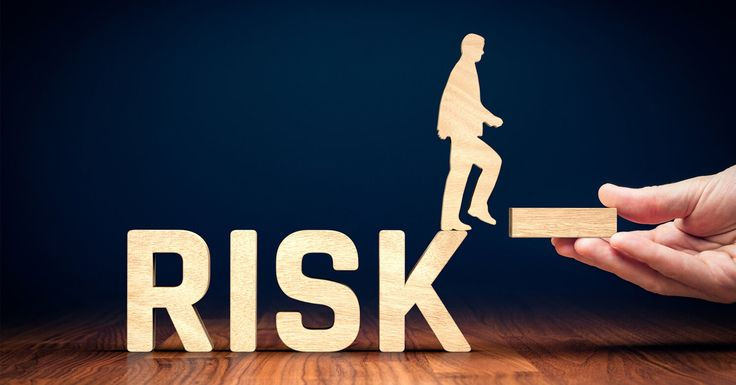
This article explores the transformative role of data mining in ERM, demonstrating how it helps companies predict and manage risks more accurately. We'll dive into how it’s used in real-world scenarios, the tools that make it possible, and the essential role of organizational training in ensuring effective implementation.
Impact of Data Mining on Enterprise Risk Management Today
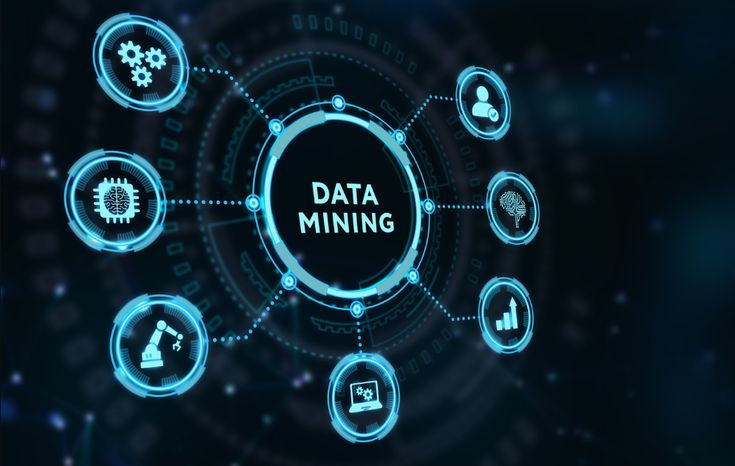
Integrating data mining into ERM is changing the way organizations approach risk. Traditionally, risk management relied on past data and qualitative insight to anticipate issues. Data mining has allowed organizations to move from a reactive approach to a proactive one. By sifting through vast amounts of structured and unstructured data, data mining empowers companies to:
Improve risk prediction: With its ability to detect patterns and anomalies in real-time and historical data, data mining helps identify potential risks before they emerge.
Enhance decision-making: Data-driven insights give leaders a clearer picture of potential threats, informing more accurate and effective risk mitigation strategies.
Increase efficiency: Automated data mining cuts down on time spent in manual risk analysis, freeing up resources for strategic planning and other critical tasks.
Effects of Data Mining on Risk Identification and Mitigation
Data mining now has a direct impact on the risk management process, especially in the areas of risk identification and mitigation. Let’s look at this in more details.
1. Risk Identification
Data mining offers the unique ability to detect risks that would otherwise go unnoticed. By analyzing extensive datasets, such as customer transaction logs, companies can identify patterns that may signal financial or reputational risks. For instance, unusual transaction patterns can alert to potential fraud, while trend analysis can reveal emerging market risks.
2. Risk Mitigation
Once risks are identified, data mining aids in developing focused mitigation strategies. Predictive models can assess the likelihood of future incidents, enabling companies to allocate resources effectively. For instance, predictive analytics helps forecast equipment failures in manufacturing, allowing for preventive maintenance rather than costly emergency repairs.
Applications of Data Mining in ERM Practices
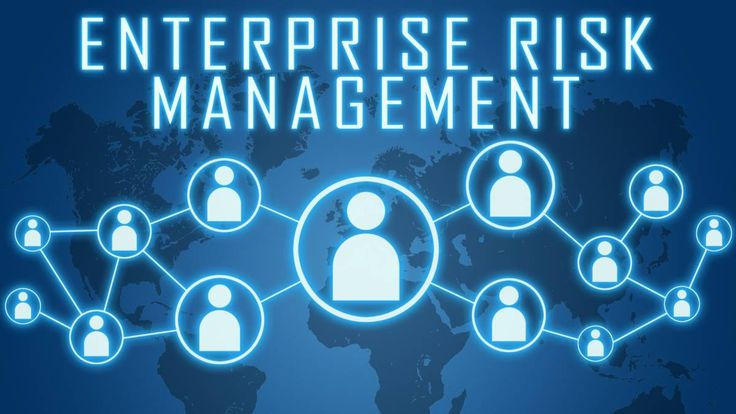
Data mining is applied across several areas of risk management, each serving a unique purpose in minimizing enterprise risk.
1. Credit Risk Management
In finance, data mining helps develop robust credit scoring models. By analyzing variables like a customer’s credit history, income, and spending behavior, banks can estimate the likelihood of loan default, helping them make smarter lending decisions. Lending platforms like Lending Club use data mining algorithms to assess borrower creditworthiness, analyzing a variety of data points, including social media behavior and transaction history, for more accurate evaluations.
2. Fraud Detection
Fraud detection is another critical application of data mining. Using anomaly detection, it identifies irregularities that may suggest fraudulent behavior, such as a spike in the number of transactions in a short timeframe. Such indicators prompt further investigation, reducing potential financial losses. Banks like JPMorgan Chase leverage data mining to analyze transaction patterns and detect unusual activity, effectively reducing the financial impact of fraud.
3. Operational Risk
In sectors like manufacturing and supply chain management, data mining helps detect inefficiencies, predict equipment failures, and enhance process optimization. Predictive maintenance uses historical data to forecast failures, minimizing downtime and increasing overall efficiency. Manufacturers like General Electric employ data mining for predictive maintenance. By analyzing sensor data from equipment, they can foresee potential failures and avoid costly downtime.
4. Market and Strategic Risk
Data mining provides insights into market trends by analyzing data from stock prices, sales patterns, and customer feedback. This helps companies anticipate shifts in the market, allowing them to adapt strategies proactively rather than reactively.
Tools Used in Data Mining for ERM
A range of tools facilitate data mining within ERM frameworks, making it accessible across various business functions.
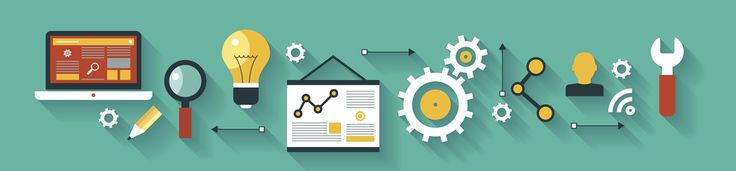
1. SQL (Structured Query Language)
SQL is widely used in relational databases for querying and analyzing data, allowing ERM teams to uncover trends and outliers in large datasets.
2. Python and R
Python and R are popular programming languages for data analysis. With libraries such as Pandas and NumPy for Python and ggplot2 for R, these languages offer powerful tools for preprocessing data, performing statistical analysis, and building predictive models.
3. Power BI
Power BI enables data visualization, allowing organizations to identify risks interactively via dashboards and reports. This tool makes complex data accessible and actionable for decision-makers.
4. SAS (Statistical Analysis System)
SAS is known for its advanced analytics capabilities, used frequently in financial risk management to develop intricate models for scenario analysis and predictive insights.
Advantages of Data Mining in ERM
Proactive Risk Management: Data mining enables early detection of risks, allowing for preventive measures.
Efficiency Gains: Automating data analysis reduces time and effort in manual risk assessments.
Enhanced Accuracy: Data mining minimizes human bias and errors by using objective data.
Cost Savings: Early risk identification helps reduce potential losses and risk management costs.
Disadvantages of Data Mining in ERM
Data Quality Challenges: Data mining’s effectiveness hinges on accurate and complete data. Poor data quality can lead to misleading results.
Implementation Costs: Establishing a data mining infrastructure can be expensive, particularly for smaller firms.
Complexity: Effective data mining requires specialized skills, which may be lacking within some organizations.
Privacy Concerns: Personal data use, especially in fraud detection or credit risk, raises ethical and regulatory considerations.
Why Organizations Should Implement Data Mining in ERM
Implementing data mining in ERM is not merely a technological upgrade but a strategic necessity. Given the volume and complexity of data in today’s business environment, manual risk management methods are insufficient. Data mining transforms raw data into actionable insights, helping organizations gain a deeper understanding of risks, both current and emerging.
By adopting data mining in ERM, companies can:
Minimize Financial Losses: Early detection and mitigation reduce the severity of risk impacts.
Gain Competitive Advantage: A data-driven approach enables better decision-making, setting companies apart in the marketplace.
Improve Regulatory Compliance: Data mining helps track and monitor compliance requirements continuously, reducing regulatory risks.
The Importance of Training ERM Teams in Data Mining
Data mining’s success in ERM relies on a well-trained workforce. Organizations must invest in training programs to equip risk management teams with data mining skills, from data collection and cleaning to analysis and ethical considerations. Effective training ensures that teams can interpret data insights and apply them practically, making the most of data mining technology.
Conclusion
Data mining is revolutionizing Enterprise Risk Management by helping organizations identify, evaluate, and address risks more efficiently than ever. Through its capability to analyze extensive datasets and provide predictive insights, data mining strengthens a company’s ability to respond to risks proactively. However, successful integration of data mining requires a skilled workforce, underscoring the importance of training for risk management teams.
By embracing data mining and equipping staff with the skills to utilize it, organizations can build a robust, forward-thinking approach to managing risks in today’s data-intensive business environment. Reach out to TA INSIGHT HUB today to get started.
Comentarios