UNDERSTANDING AUGMENTED ANALYSIS
- Jennifer
- Oct 21, 2024
- 4 min read
Updated: Nov 6, 2024
The term Augmented Analysis might be unfamiliar to some, but it is rapidly becoming a trend and key component of modern Business Intelligence (BI). This article aims to explain what augmented analysis (AA) is, how it works, and how businesses can integrate it into their BI systems.
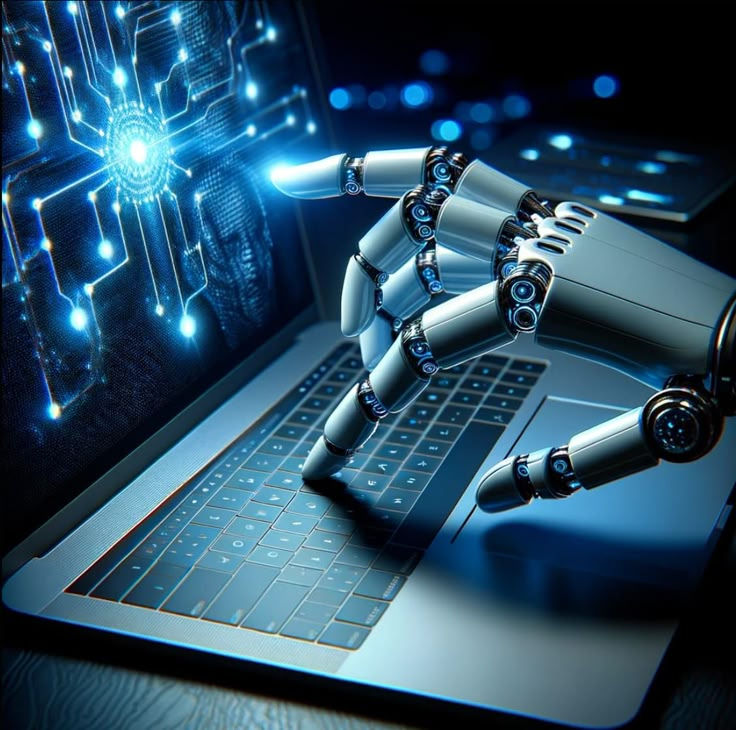
What is Augmented Analysis?
Augmented analysis represents a new wave in BI, as more organisations adopt it to enhance data analysis for improved outcomes. This trend integrates artificial intelligence (AI) and machine learning (ML) with traditional analytics to streamline and automate processes like data preparation, insight generation, and decision-making.
In simple terms, augmented analytics leverages AI and ML to simplify data analysis, uncover hidden patterns, and generate insights faster and more accurately than manual methods. While it enhances the analytical process and reduces time, it does not fully replace human decision-making, as the human element remains vital for interpreting and applying insights to real-world business scenarios. Augmented analysis functions much like a virtual data scientist, carrying out analytical tasks and operationalising data-driven findings.
How Does Augmented Analysis Work?
AA automates several stages of the data analytics process, including data preparation, identifying patterns, and communicating findings. It relies on AI, ML, natural language processing (NLP), and natural language generation (NLG) to automate the analysis.
Here’s a breakdown of the process:
Data Preparation: Automated tools clean and organise data, eliminating repetitive manual tasks.
Insight Generation: AI algorithms identify trends, correlations, and outliers, often providing insights that would be difficult to detect manually.
Natural Language Processing (NLP): NLP allows users to interact with data using everyday language, making it accessible to non-technical users. Insights are presented in clear, visual formats, aiding quick decision-making.
By automating tedious tasks, augmented analytics frees up analysts to focus on more complex problems, while enabling non-technical users to explore data without needing expert knowledge.
Key Benefits of Augmented Analysis
Empowering Non-Technical Users: AA extends advanced analytics capabilities to users who may not have deep technical expertise, enabling them to perform sophisticated data analysis with ease.
Improving Accuracy: It eliminates human biases in data interpretation and highlights previously unidentified growth opportunities.
Enhanced Resource Utilisation: Analysts can focus on high-value tasks, while routine tasks are automated. This leads to greater efficiency in the workplace.
Saving Time: Traditional BI platforms require time-consuming manual data preparation, whereas AA automates these processes, significantly reducing time spent on analysis.
Cost-Effective: By automating key tasks, AA reduces costs associated with data cleaning, reporting, and analysis.
Challenges of Augmented Analytics
While AA brings many advantages, its implementation is not without challenges. Data quality and governance are critical for ensuring that AI models produce accurate insights. Without clean, structured data, even the most sophisticated algorithms can generate misleading results. Additionally, businesses need to invest in user training to ensure staff understand and effectively utilise the technology.
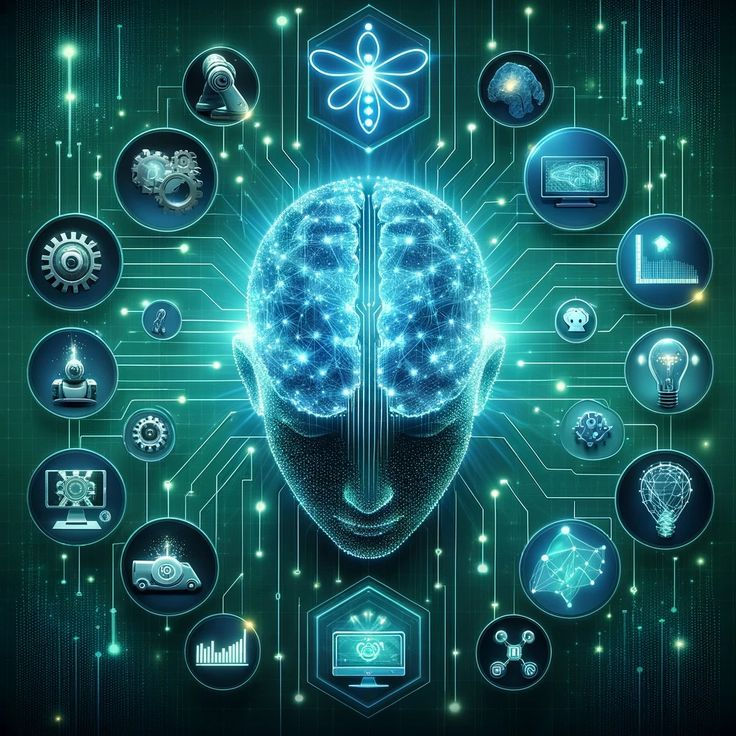
Applications Across Industries
Augmented analytics has practical applications across various sectors:
Healthcare: It facilitates personalised care by analysing patient data, accelerating disease diagnosis, and improving treatment recommendations. It also supports efficient drug development and reduces long-term costs.
Finance: In finance, AA enhances fraud detection, improves forecasting, and enables continuous auditing, helping businesses make informed decisions and reduce operational risks.
Logistics and Transportation: AA optimises supply chains by predicting transportation delays and improving decision-making, leading to cost savings and increased efficiency.
Manufacturing: It streamlines manufacturing processes, improves decision-making through real-time data visualisation, and enables predictive maintenance to minimise downtime.
Examples of Augmented BI Tools
Several platforms have incorporated AA features to automate key analytics tasks:
Decooda: Uses AI to automate data cleaning, reorganisation, and transformation.
IBM InfoSphere Advanced Data Preparation: Provides ML-driven recommendations for data preparation via automated dashboards.
Qlik Technologies & Tableau: Utilise AI to recommend visualisations and identify variable correlations. They support NLP, allowing users to interact with data in conversational language.
DataRobot, H2O.ai, Anodot: Provide AutoML tools for model testing and monitoring.
SAP’s Smart Discovery: Assists decision-making by generating insights via Natural Language Processing (NLP), and Natural Language Generation (NLG).
Microsoft Power BI: Recently integrated AI-driven insights and predictive analytics through Azure Machine Learning.
Key Differences to Understand
Augmented Analytics vs. Automation: Automation performs repetitive tasks, while augmented analytics enhances the data analysis process by automating insight generation through AI and ML.
Predictive Analytics vs. Augmented Analytics: Predictive analytics focuses on forecasting outcomes using historical data, while AA encompasses a wider range of capabilities, including data preparation and NLP.
Augmented Analytics vs. Self-Service Analytics: Self-service analytics allows non-technical users to explore data independently. AA takes this further by automating complex analysis, making it easier for non-experts to derive insights.
Future Outlook
The future of BI is firmly rooted in augmented analytics. As AI and ML continue to evolve, AA will offer deeper insights and allow businesses to anticipate trends more accurately. Its ability to automate routine tasks and generate precise predictions will play a vital role in driving data-driven decision-making across industries.
Conclusion
To thrive in today’s competitive market, businesses must leverage data effectively. BI and analytics are central to this process, and augmented analysis represents the next step in the evolution of data analysis. By incorporating AI and ML into BI, AA enables organisations to extract meaningful insights more efficiently, reducing the complexity of traditional analytics. Ultimately, it empowers users at all levels to make data-driven decisions that drive business success.
Comments