A/B Testing - A Case Study on Marketing Conversion Rates
- Abdulazeez Abdullah Temitope
- Jul 30, 2024
- 2 min read
Updated: Nov 6, 2024

A/B testing is a powerful statistical technique used in data-driven decision-making. It involves creating two versions (A and B) of a webpage, email, product or advertisement and randomly assigning users to experience one version. By comparing the performance metrics of these variants, businesses can make informed decisions about which version drives better results. This methodical approach is crucial for optimizing marketing campaigns, improving website user & product experience, and enhancing overall business efficiency.
Why A/B Testing
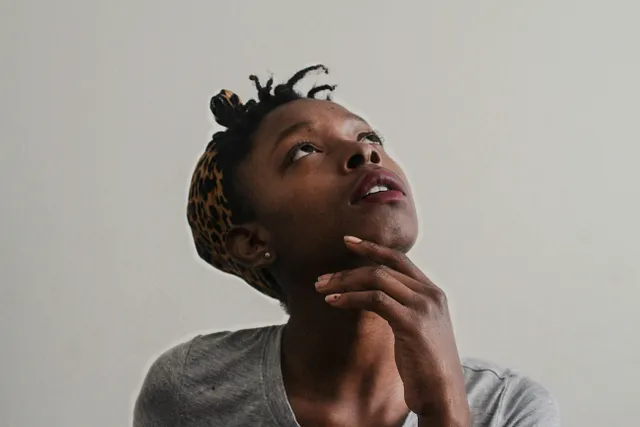
A/B testing offers several key benefits:
Data-driven decisions: It eliminates guesswork by providing concrete data on what works best.
Improved performance: By identifying the best-performing variations, you can optimize your website, product or app for maximum impact.
Increased conversions: A/B testing can lead to higher conversion rates, whether it's sales, sign-ups, or other desired actions.
Better understanding of users: By analyzing user behavior, you can gain valuable insights into user preferences and needs.
Continuous improvement: A/B testing is an ongoing process that allows you to continually refine your website, product or app based on user feedback.
Case Study: Advertisement vs. Public Service Announcements

An organization conducted a case study to evaluate the performance of advertisements (ads) and public service announcements (PSAs) in driving conversions. The primary objective was to identify which format generated higher conversion rates.
Insights

Data analysis revealed several key findings:
Overall Performance: Ads generally outperformed PSAs in terms of conversion rates.
Peak Performance: The most effective days for both ads and PSAs were Mondays and Tuesdays.
Ad Volume: A smaller number of ads (less than 100) tended to yield higher conversion rates.
Optimal Timing: Both ads and PSAs achieved the best results when displayed between 12:00 PM and 4:00 PM.
Statistical Analysis

Given the relatively small sample size, it's essential to determine if the observed differences in conversion rates between ads and PSAs are statistically significant or simply due to random chance. A T-test will be employed to analyze this. We hypothesise that the differences between the two target groups are not random, making the null hypothesis the opposite.
T-test in excel
T-test in python
The results from both platforms (Excel and Python) consistently indicated that the observed differences were statistically significant, supporting the conclusion that ads were more effective than PSAs in driving conversions. The consistency of the results on both tools also shows that irrespective of the tool you use, you will arrive at the same result. Download the results and data here.
The findings of this A/B test provide compelling evidence that advertisements outperform public service announcements in terms of conversion rates. The statistical significance of the results, as determined by both Excel and Python T-tests, reinforces the reliability of these conclusions.
By understanding the nuances of A/B testing, organizations can harness its power to optimize various aspects of their operations. Whether it's refining website design, enhancing email marketing campaigns, or improving the efficacy of advertising, A/B testing offers a systematic approach to data-driven improvement. As technology continues to advance, the potential applications of A/B testing are expanding, making it an indispensable tool for businesses seeking to stay ahead of the competition.
It's crucial to note that while this study provides valuable insights, further research with larger sample sizes and additional variables could offer an even more comprehensive understanding. Continuous A/B testing and optimization are essential for sustained success.
Comments